Understanding Artificial Intelligence and Its Evolutions
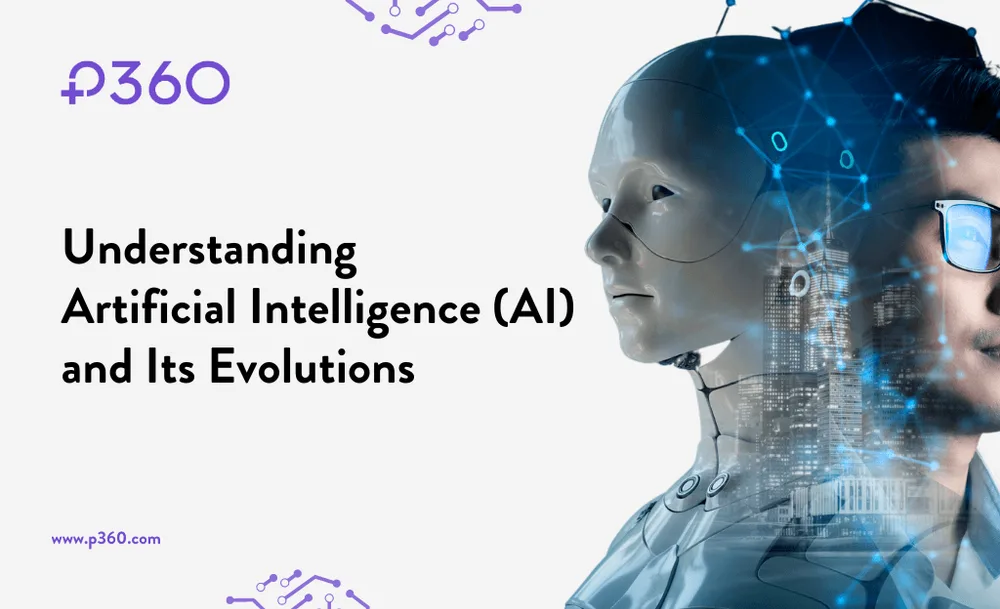
by Kimberly Gregorio | Last Updated: May 24, 2023 | 1 min read
Unless you’ve been living on a remote island with no Internet the last few years, chances are you’ve come across the words "Artificial Intelligence," or “AI.” Once the thing of books and movies, AI has gained widespread popularity and has become an integral part of our everyday lives. From voice assistants like Siri and Alexa to self-driving cars and personalized recommendations, AI has revolutionized various industries. But what exactly is AI? In this article, we will explore the concept of AI, its underlying principles, and its impact on society.
What is Artificial Intelligence?
At its core, AI is simply the simulation of human intelligence through computer technology. It is a way to train machines to do what humans do, whether it is learning, reasoning, or self-correcting. AI can come in many forms, from autonomous robots to intelligent algorithms. It can be further categorized into three main types: narrow, general and super.
The first type, Narrow AI, also known as weak or applied AI, focuses on one particular task. Examples of Narrow AI include chatbots, image recognition software, and recommendation systems used by businesses like Amazon and Netflix. It is designed to do one thing well and does not possess the capability for generalized human-like intelligence.
General or strong AI, on the other hand, is intended to replicate human-level intelligence and adaptability. These AI systems have a wide range of abilities and can perform various tasks comparable to human intelligence. However, General AI is still very much a work in progress and is yet to reach human levels of reasoning and decision-making.
Finally, Super AI is the theoretical next level of AI and is yet to be achieved. It's an AI that exceeds human intelligence in all aspects and can solve problems beyond our understanding. While this future AI may seem far-fetched, the strides we are making in AI could get us there eventually.
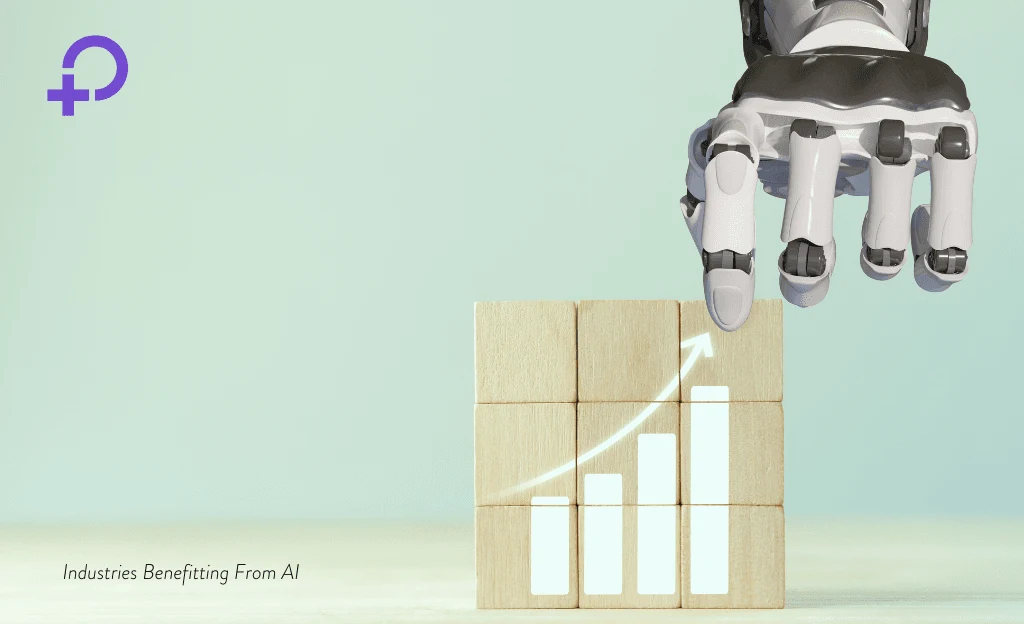
Industries Benefitting From AI
AI is transforming industries and businesses in unimaginable ways. Its capabilities are vast and its applications seem almost limitless. It is revolutionizing industries from healthcare and finance to customer service, and even the automotive industry, by providing predictive and real-time insights, automating repetitive tasks, and allowing humans to focus on more complicated endeavors. Here’s a deeper look at some of the industries AI is changing:
-
AI In Healthcare: AI-powered platforms have shifted the healthcare industry’s focus from reactive to preventative care. Patients can get a more accurate diagnosis and personalized treatment plan with the help of AI systems like IBM’s Watson. AI algorithms are trained on huge volumes of patient data, allowing doctors to predict the outcome of certain treatments better and avoid misdiagnoses. In drug discovery, AI can analyze millions of chemicals at scale faster and cheaper than traditional methods. Insilico Medicine, a startup, uses AI to create novel peptides that address the leading cause of death in elderly patients. AI’s impact goes beyond predictive analytics as it can perform tedious administrative tasks like appointment scheduling, freeing up doctors, and allowing them to focus more on their patients.
-
AI in Finance: AI is transforming the way we bank and trading experience. In financial services, AI-powered chatbots are transforming customer service by providing tailored real-time assistance, decreasing waiting times, and improving customer experience. The algorithms can detect unusual activities, report fraud, and assess risks accurately, thereby reducing the chances of financial fraud. In investment banking and trading, AI algorithms with natural language processing capabilities analyze news, economic indicators, and market trends data to determine trading biases and generate trading algorithms that outperform human traders.
-
AI in Customer Service: AI-powered chatbots are automating the customer service industry by providing instant responses and personalized support to customer queries. They can understand complex customer service requests and offer timely solutions. AI chatbots free customer service teams to focus on complicated queries they’re the best equipped to resolve. Chinese e-commerce giant Alibaba’s Tongyi Qianwen AI-powered virtual store assistant can hold meaningful conversations with customers, helping them navigate and make purchases on the platform. AI’s impact goes beyond chatbots as facial recognition technology deployed to hotels and airports allows customers to check-in and board planes with minimal human interaction.
-
AI in the Automotive Industry: AI is revolutionizing the automotive industry through self-driving cars, enhancing road safety and environmental sustainability. Self-driving cars have the potential to signal the end of road accidents, improve traffic, and reduce road congestion. AI-powered algorithms can collect data on external conditions, other cars, and road users to ensure safety. AI’s impact goes beyond self-driving cars as AI-powered computer vision and analytics technology also enables traffic management systems that optimize transportation efficiency.
What About Machine Learning and Neural Networks?
The terms Artificial Intelligence, Machine Learning and Neural Networks are often used interchangeably, and it’s not uncommon for people to confuse the technologies with each other. But while they are closely related, each one has unique features that allow machines to learn and evolve in different ways. Knowing the differences between them can help businesses determine which technology is best suited for their objectives.
Machine Learning (ML) is a subfield of AI that involves teaching machines to learn on their own by analyzing data and making predictions. Essentially, ML algorithms use statistical techniques to identify patterns in data, which they then use to make predictions about new data. The more data an ML algorithm is trained on, the better it becomes at making predictions. Some of the most common types of ML algorithms include regression, classification, clustering, and deep learning.
Neural Networks are a subset of ML, but they are designed to mimic the human brain’s structure and functioning. They consist of a large number of interconnected nodes, each of which processes information and passes it on to other nodes. Neural networks can learn on their own by adjusting the strength of the connections between nodes, which allows them to identify patterns in data and make predictions. One of the most common types of neural network is the Convolutional Neural Network (CNN), which is widely used in image recognition.
So, in a nutshell: Artificial Intelligence is a broad category consisting of many different technologies that allow computers to learn, make decisions and perform tasks that typically require human-like intelligence. Machine Learning is a subfield of AI that focuses on algorithms that can learn from data and make predictions. And Neural Networks are a subset of ML that are designed to mimic the human brain, allowing them to learn from data and make predictions.
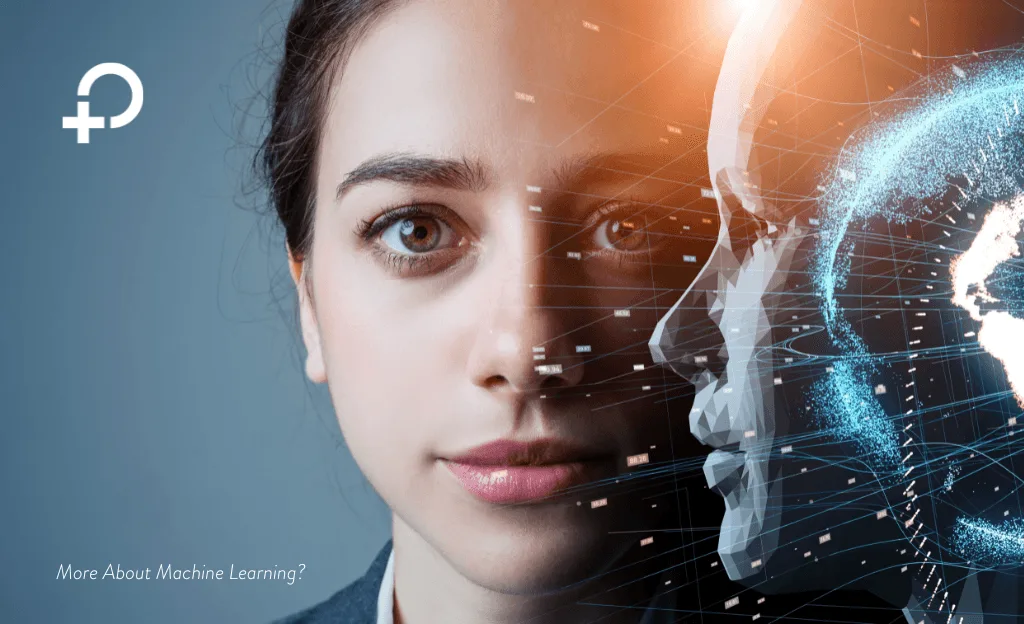
More About Machine Learning?
At its core, machine learning revolves around the idea of training a model using a large dataset to recognize patterns and make accurate predictions or decisions based on new, unseen data. This process involves several key components:
-
Data - Machine learning algorithms require a substantial amount of relevant and representative data to learn from. This data can include structured information, such as numerical values, and unstructured data like text, images, or audio.
-
Features - Features are specific characteristics or attributes extracted from the data that the model uses to understand patterns. Selecting the right features is crucial as it directly influences the model's performance.
-
Algorithms - Machine learning algorithms are mathematical models that analyze data, identify patterns, and make predictions or decisions. These algorithms can be categorized into different types, including supervised learning, unsupervised learning, semi-supervised learning, and reinforcement learning, each with its own approach to learning from data.
-
Training - During the training phase, the machine learning model learns from the labeled or unlabeled data provided. It adjusts its internal parameters and optimizes its performance by minimizing errors or maximizing a specific objective function.
-
Evaluation and Testing - Once the model is trained, it needs to be evaluated using a separate set of data called the test set. This evaluation helps assess the model's performance, such as its accuracy or error rate. If the performance is satisfactory, the model can be deployed for making predictions or decisions on new, unseen data.
Machine learning has numerous applications across various fields. For instance, image and speech recognition, where ML models are used to develop systems that can accurately recognize and classify images, identify objects or faces, and transcribe speech into text. There is also Natural Language Processing (NLP), where ML algorithms enable machines to understand and interpret human language. This is utilized in applications such as chatbots, sentiment analysis, and language translation.
ML algorithms also power recommender systems, which are recommendation engines that suggest products, movies, or music based on a user's preferences and behavior. These models can also be used for fraud detection to find anomalies and patterns in large datasets, helping identify fraudulent transactions or activities. ML even plays a crucial role in self-driving cars by enabling them to perceive their surroundings, make decisions, and navigate safely.
As ML continues to evolve, its potential for innovation and its ability to automate complex tasks make it a key component of the AI ecosystem.
More About Neural Networks?
The basic building block of a neural network is an artificial neuron, also called a perceptron. It receives input signals, applies weights to those signals, performs a mathematical operation on them, and produces an output. These artificial neurons are organized into interconnected layers, forming the structure of the neural network. A typical neural network consists of three main types of layers:
-
Input Layer: This is the initial layer of the network where the input data is fed. Each neuron in the input layer represents a feature or attribute of the input data.
-
Hidden Layers: Hidden layers are intermediate layers between the input and output layers. They perform complex computations and transformations on the input data. Neural networks can have multiple hidden layers, each consisting of several neurons. The depth and width of the hidden layers determine the network's capacity to learn and represent complex patterns.
-
Output Layer: The output layer is the final layer of the network, producing the predicted or desired output based on the processed information from the hidden layers. The number of neurons in the output layer depends on the nature of the task. For example, in a classification problem with multiple classes, each neuron may represent the probability of belonging to a particular class.
Neural networks learn by adjusting the weights associated with the connections between neurons during a training process called backpropagation. Backpropagation involves comparing the network's predicted output with the desired output and updating the weights to minimize the error or loss function. This iterative process continues until the network achieves the desired level of accuracy or convergence.
It is also important to note that there are several types of neural networks, including:
-
Deep Neural Networks, which are neural networks with multiple hidden layers, allowing them to learn hierarchical representations of the data. “Deep learning” leverages the power of deep neural networks to extract intricate features and patterns from complex datasets, enabling breakthroughs in areas such as image recognition, natural language processing, and speech recognition.
-
Convolutional Neural Networks (CNNs), which are widely used for tasks like image classification, object detection, and facial recognition.
-
Recurrent Neural Networks (RNNs) and Transformers, which are employed in language modeling, machine translation, sentiment analysis, and speech recognition.
Like AI and ML, there are various applications for neural networks. For example, deep neural networks are essential for perception, decision-making and control in self-driving cars. They can also assist in disease detection, medical imaging analysis and drug discovery.
Businesses are also using neural networks to predict customer behavior and tailor marketing strategies around their habits. This has allowed businesses to offer targeted advertising by accurately predicting customer behaviors based on their purchasing history, online activity and demographic information. Neural networks have even become a critical tool in the manufacturing industry, where they are used for predictive maintenance. They can be trained using data from manufacturing equipment and machines to detect and forecast potential failures, faults, and equipment breakdown.
Ethical Considerations
AI, ML and neural networks have opened up new opportunities in numerous fields of business. However, as these technologies grow more sophisticated, they also raise significant ethical concerns that need to be carefully addressed. From concerns over privacy and bias, to job displacement and accountability, there are a range of issues that business leaders need to consider as they explore the benefits of AI and its related technologies.
-
Privacy: Privacy concerns are one of the most pressing ethical considerations related to AI and ML. These technologies often involve collecting vast amounts of personal data, which can be used to make powerful predictions about consumer behavior. While these insights can be valuable for businesses, they may also create significant risks for individuals. Recent high-profile data breaches have highlighted the need for improved data protection measures, and it is increasingly important for businesses to take a proactive approach to data security. This may involve implementing advanced encryption systems and investing in high-level cybersecurity talent.
-
Bias: Bias is another important ethical issue associated with AI and related technologies. Such technologies often rely on large datasets to identify patterns and make predictions, but these datasets may reflect problematic biases. For example, if an AI system relies on data from a predominantly white male population, it may be more likely to produce biased results that fail to account for the perspectives and behaviors of other demographic groups. To avoid these pitfalls, it is essential that businesses take steps to create more inclusive data sets, and to test their algorithms for potential bias.
-
Job Displacement: The rapid growth of AI and related technologies also raises important questions around job displacement. As machines become more capable of performing complex tasks, it is possible that some jobs will be rendered obsolete. While new types of jobs will emerge to fill the gap, many workers may still face significant challenges in adapting and finding new roles. As a result, it is important for businesses to be mindful of the potential impacts of AI on their workforce, and to support their employees through times of transition.
-
Accountability: Accountability is a final ethical consideration that must not go under the radar when dealing with AI. As machines make more decisions for us, it becomes increasingly difficult to determine who is responsible for actions that are guided by these decisions. The issue of accountability strikes at the very heart of our social contract, and it is vital for businesses to address it effectively. Developers and users must ensure that AI systems are transparent and accountable, and that they can provide clear explanations for the decisions they make.
That is why advanced algorithms must be designed to uphold ethical standards, ensuring fairness, transparency and accountability.
Explore More Relevant Articles on P360
- Diversity Alliance for Science (DA4S) Selects P360 for Eight That Innovate Program
- Adding Data Integrity to the HCP Moral Code, The Key to HCP Communication and Trust
- ZING and Swittons are Now SOC 2 Type II Compliance Certified
- P360’s Data and Analytics Platform BirdzAI Named in Recent Gartner Research Note
- P360 is a sample vendor in Gartner® Hype Cycle™ 2022 report
Final Thoughts
Artificial Intelligence, Machine Learning and Neural Networks are transforming the world as we know it, revolutionizing industries, enhancing productivity, and improving our daily lives. From healthcare marketing to finance, transportation to customer service, the potential of these technologies is immense. By understanding the concepts, applications and ethical implications of AI, businesses can leverage its power responsibly for the betterment of society.